Is yours big enough?
This blog was prompted by a question and comment from a client, who asked “Given we provided a database of 6,320 contacts, and you received completed questionnaires from 180 respondents rating our firm, is that enough? The sample seems small compared to the size of the database.”
If I rephrase our client’s words, what they are asking are two questions: [1] How do we judge what an adequate sample size is? and [2] what role the size of the population we are sampling from has in deciding whether our sample is adequate or not?
Confidence intervals
We are interested in sample size because it indicates how confident we should be in our survey estimate. When we are talking about confidence in a statistical sense, we mean confidence intervals. So let’s start with here. Many researchers will be familiar with this equation for a 95% confidence interval for a survey proportion p:
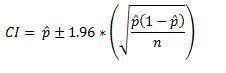
In this equation, the p can be any proportion estimate from your survey…”the proportion of respondents who are aware of your firm”…”the proportion of respondents who would consider your firm”...or “the proportion of a firm’s respondents from Western Australia”, for example.
If our survey estimate is p=50% and our confidence interval is (40%, 60%), we often hear people interpret this as “We are 95% confident that the true population proportion lies within the confidence interval of 40% to 60%”. This wording is too loose and not correct. The theory behind the confidence interval actually says that if we were to repeat the same survey 100 times, and then for each of those 100 surveys we were to calculate a proportion and the 95% Confidence Interval for that proportion, then the 95% confidence interval for 95 of those 100 surveys would contain the true population proportion.
While this explanation is somewhat wordy, it is the correct interpretation. Let’s look at the formula a little more closely. The part after the “±” sign is called the Margin-of-Error (MOE), which is represented as follows:

What we observe from the two formulae above is that if the MOE decreases, the Confidence Interval gets smaller and we are more confident in our estimate.
So how do we make the MOE smaller?
We can’t control the p, as this is our survey estimate, and we only know that after the fieldwork is completed.
The 1.96 is taken as given for a 95% Confidence Interval.
So the only remaining element we can change is the sample size n in the denominator.
So let’s see how the MOE changes for different values of n by using the chart below. We can see that as the sample size increases the MOE decreases. But we can also see that the decrease in the MOE gets smaller for higher sample sizes, so that the line starts to flatten out.
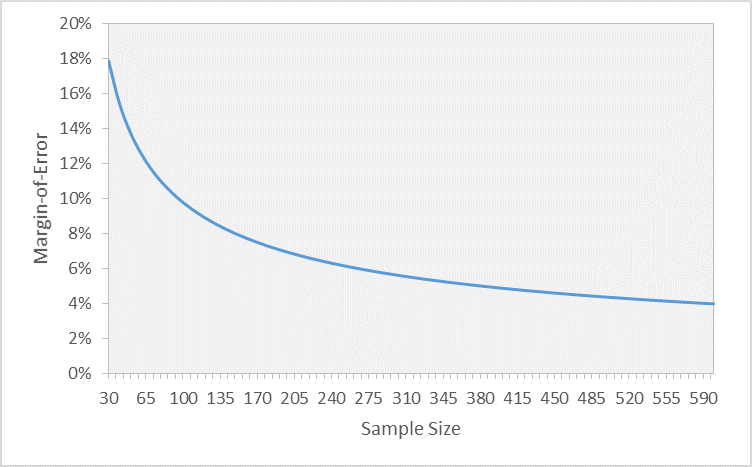
A common sample size used by researchers is n=100, and for a sample size of n=100 we have a MOE of approximately 10% from the graph on the left. This means that if we get a survey estimate that says 50% of respondents surveyed use a particular professional services firm, for example, then the confidence interval is 40%-60%.
Therefore, we are interested in the sample size mostly because it indicates how large the MOE for our survey estimate is, which in turn tells us how confident we can be in our survey estimate.
With that in mind, a higher sample size will obviously give us more confidence in our estimate, but we will need to pay the additional cost to recruit those extra respondents (and in many B2B markets like professional services they are simply not available). For many researchers, the sample size of 100 often gives a nice trade-off between having an acceptable level of confidence, i.e. a MOE of 10%, and keeping the cost of the survey under control.
Size of the population
But what about the size of the population from which we are sampling? Isn’t taking a sample size of n=100 from a population of N=1,000 people different from taking a sample size of n=100 from a population of N=10,000 people? The answer to this is Yes and No. Yes, the MOE based on a sample taken from a population of 1,000 will be different to a MOE from a sample of the same size taken from a population of 10,000. But, in most situations, there will be no practical difference between MOE from a population of 1,000 and 10,000.
To understand why this is so, let’s go back to the MOE equation. In the earlier equation I left out the part of the equation that is called the “finite-population-correction” factor (FPC) when we are sampling without replacement, which is expressed like this:
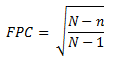
Where N is the population size, and n is the sample size.
This can be included in the MOE as follows,

What the FPC equation shows is that as the population size N increases, the sample size n and the 1 in the denominator won’t matter to the FPC, so that effectively the FPC part of the equation converges to one and doesn't affect the MOE.
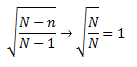
To visualise this, for a sample size of n=100, I have plotted the FPC for different sizes of the population N below. Notice that with a sample size of n=100, when the population size N is larger than 1,000 the FPC becomes almost flat and is approximately 1.

As 100/1,000=10%, this means that as long as the sample is smaller than 10% of the population being sampled, it doesn’t really matter what the size of the population is as the FPC will be approximately 1, and so doesn’t influence the MOE.
Therefore if you are a small firm and provide a database of 1,000 clients for the beatonbenchmarks, or if you are a large firm and provide a client database of 100,000 clients for the beatonbenchmarks, it doesn’t really matter, as if we take a sample of n=100 from either of these two databases the estimate will have a MOE of approximately 10%.
How do I use this to know if our sample size is sufficiently large?
This is simple, we simply solve the Confidence Interval equation for n, like this,

When using the formula before fieldwork we just set p to 0.5, as that will give you the largest possible MOE for the stated sample size.
So if we are planning a survey and we want our estimate to have a 95% confidence interval of plus or minus 8%, then we would calculate as follows…

…showing we need a sample size of 150 for a MOE of 8%.
If your database size is smallish then you can add in the FPC again check using,
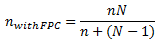
where n is the sample size from the equation without the FPC.
Therefore, if you want a MOE no larger than 8%, and you have a database of 7,000 clients, then would need a sample size of,
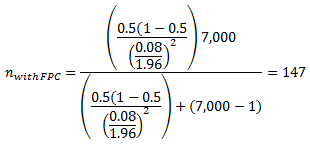
If, on the other hand, your database was of size 600, then you would need a sample size of at least 80 to maintain an MOE of approximately 10%,
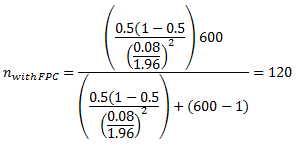
Rule of thumb

If this has all been a bit too much for you, here is a rule of thumb for approximating your MOE.
A simple approximate calculation of the MOE is shown on the left.
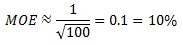
So that if you have a sample size of n=100, then your MOE should be approximately 10%.
In conclusion
In conclusion, the sample size needed depends on how confident you want to be in your estimate. If you want more confidence in your survey estimate, then sample more. Most researchers are happy with a MOE of 10%.
We also noted that the size of the population you are sampling from doesn’t really matter if the sample is less than 10% of the population being sampled, which is usually the case in most surveys.
Therefore, for the client who I alluded to in the introduction to this blog, who asked about whether their sample size of 180 from a database of 6,320 is adequate, should be happy. Their sample size of 180 give a MOE of 7.3%, which is very good, and their database size is large enough that we don't need to factor in the Finite Population Correction factor.
Author
Grant Hollings is a Research Manager at beaton.